Modélisation de la croissance du bersim (Trifolium Alexandrinum L.) et du rendement de la biomasse sous différents niveaux de stress hydrique à Tadla, au Maroc
Résumé
Ce travail compare les performances de PILOTE, un modèle opérationnel développé par IRSTA de Montpellier-France, avec celles d’un modèle plus élaboré, CropSyst, dans la simulation de croissance du bersim (Trifolium Alexandrinum L.) conduit sous différents régimes hydriques au Tadla (Maroc). L’accumulation de la matière sèche selon la loi de Beer via la radiation potentielle active interceptée (IPAR) constitue le point commun des deux modèles. Ils diffèrent par le niveau de complexité relative à la description du développement et la croissance de la culture, le principe d’absorption de l’eau par les racines et par conséquent le nombre d’input. Les deux modèles ont été calés sur le régime hydrique non limitant en 2009/10, puis validés sur les autres traitements de 2009/10 et de 2010/11. Bien que PILOTE nécessite moins de paramètres, il s’avère comparable à CropSyst dans la simulation de la biomasse et du bilan hydrique. L’utilisation d’un nombre différent de paramètres et de modules par les deux modèles testés n’a pas influencé sensiblement les résultats de la simulation. Par conséquent, dans un objectif limité à la gestion de l’eau et dans des conditions de manque de paramètres culturaux, l’utilisation de modèles plus simples devrait être encouragée.
Mots clés: Modèle de culture, bersim, PILOTE, CropSyst, stress hydrique, Maroc
Téléchargements
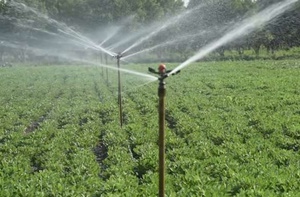
Publié-e
Comment citer
Numéro
Rubrique
Licence
Revue Marocaine des Sciences Agronomiques et Vétérinaires est mis à disposition selon les termes de la licence Creative Commons Attribution - Pas d’Utilisation Commerciale - Partage dans les Mêmes Conditions 4.0 International.
Fondé(e) sur une œuvre à www.agrimaroc.org.
Les autorisations au-delà du champ de cette licence peuvent être obtenues à www.agrimaroc.org.