Évaluation du modèle AquaCrop dans la simulation de la croissance et du rendement du blé dur (Triticum aestivum L.) sous différents régimes hydrologiques au Tadla- Maroc
Résumé
Les modèles simulant l’effet de l’eau sur le rendement des cultures peuvent être des outils utiles pour améliorer la gestion de l’eau et optimiser l’efficience de son utilisation. Dans cette étude, AquaCrop a été évalué pour la variété de blé dur (Triticum aestivum L.) Karim au Tadla. AquaCrop simule la production de biomasse proportionnellement à la transpiration de la plante via un coefficient de productivité de l’eau. Le modèle a été calé sur le régime hydrique non limitant en 2014/15 puis validé sur les autres traitements de 2014/15 et 2015/16. Les résultats ont montré que le modèle permet d’excellentes simulations du taux de couverture du sol, la biomasse et le rendement grain. En combinant tous les traitements, la relation entre le rendement grain observé et simulé a un R2 de 0,79, une erreur quadratique moyenne de 1,01 t ha-1 et un coefficient d’efficacité de 0,68. Le modèle prédit globalement la tendance du stock hydrique du sol. En conséquence, AquaCrop peut être un outil précieux pour simuler le rendement grains du blé, compte tenu en particulier du nombre relativement faible de données d’entrée. Cependant, la performance du modèle doit être évaluée dans un large éventail de conditions.
Mots clés: Modèle de culture, blé dur, AquaCrop, stress hydrique, Maroc
Téléchargements
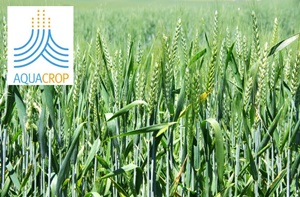
Publié-e
Comment citer
Numéro
Rubrique
Licence
Revue Marocaine des Sciences Agronomiques et Vétérinaires est mis à disposition selon les termes de la licence Creative Commons Attribution - Pas d’Utilisation Commerciale - Partage dans les Mêmes Conditions 4.0 International.
Fondé(e) sur une œuvre à www.agrimaroc.org.
Les autorisations au-delà du champ de cette licence peuvent être obtenues à www.agrimaroc.org.